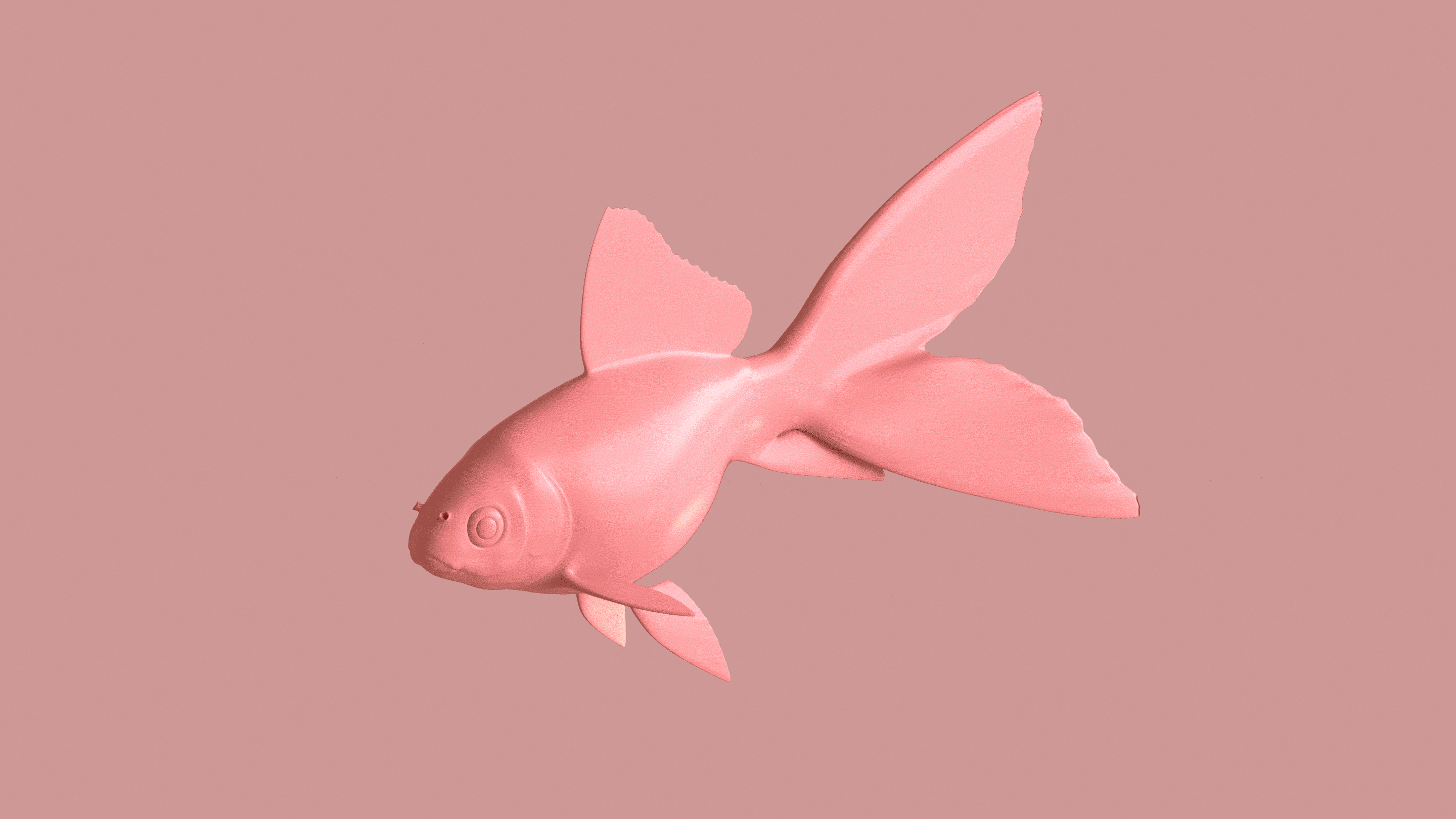
Some neuroscientists favor a predictive coding explanation for how the brain works, in which perception may be thought of as a “controlled hallucination.” This theory emphasizes the brain’s expectations and predictions about reality rather than the direct sensory evidence that the brain receives. Credit: Zolloc for Quanta Magazine.
In mid-2018, the artificial intelligence company DeepMind introduced new software that can take a single image of a few objects in a virtual room and, without human guidance, infer what the three-dimensional scene looks like from entirely new vantage points. Given just a handful of such pictures, the system, dubbed the Generative Query Network, or GQN, can successfully model the layout of a simple, video game-style maze.
There are obvious technological applications for GQN, but it has also caught the eye of neuroscientists, who are particularly interested in the training algorithm it uses to learn how to perform its tasks. From the presented image, GQN generates predictions about what a scene should look like — where objects should be located, how shadows should fall against surfaces, which areas should be visible or hidden based on certain perspectives — and uses the differences between those predictions and its actual observations to improve the accuracy of the predictions it will make in the future. “It was the difference between reality and the prediction that enabled the updating of the model,” said Ali Eslami, one of the project’s leaders.
According to Danilo Rezende, Eslami’s co-author and DeepMind colleague, “the algorithm changes the parameters of its [predictive] model in such a way that next time, when it encounters the same situation, it will be less surprised.”
Neuroscientists have long suspected that a similar mechanism drives how the brain works. (Indeed, those speculations are part of what inspired the GQN team to pursue this approach.) According to this “predictive coding” theory, at each level of a cognitive process, the brain generates models, or beliefs, about what information it should be receiving from the level below it. These beliefs get translated into predictions about what should be experienced in a given situation, providing the best explanation of what’s out there so that the experience will make sense. The predictions then get sent down as feedback to lower-level sensory regions of the brain. The brain compares its predictions with the actual sensory input it receives, “explaining away” whatever differences, or prediction errors, it can by using its internal models to determine likely causes for the discrepancies. (For instance, we might have an internal model of a table as a flat surface supported by four legs, but we can still identify an object as a table even if something else blocks half of it from view.)
The prediction errors that can’t be explained away get passed up through connections to higher levels (as “feedforward” signals, rather than feedback), where they’re considered newsworthy, something for the system to pay attention to and deal with accordingly. “The game is now about adjusting the internal models, the brain dynamics, so as to suppress prediction error,” said Karl Friston of University College London, a renowned neuroscientist and one of the pioneers of the predictive coding hypothesis.
Over the past decade, cognitive scientists, philosophers and psychologists have taken up predictive coding as a compelling idea, especially for describing how perception works, but also as a more ambitious, all-encompassing theory about what the entire brain is doing. Experimental tools have only recently made it possible to start directly testing specific mechanisms of the hypothesis, and some papers published in the past two years have provided striking evidence for the theory. Even so, it remains controversial, as is perhaps best evidenced by a recent debate over whether some landmark results were replicable.
Coffee, Cream and Dogs
“I take coffee with cream and ____.” It seems only natural to fill in the blank with “sugar.” That’s the instinct cognitive scientists Marta Kutas and Steven Hillyard of the University of California, San Diego, were banking on in 1980 when they performed a series of experiments in which they presented the sentence to people, one word at a time on a screen, and recorded their brain activity. Only, instead of ending with “sugar,” when the last word popped into place, the sentence read: “I take coffee with cream and dog.”
The researchers observed a greater brain response when the study’s subjects came across the unexpected word “dog,” characterized by a specific pattern of electrical activity, known as the “N400 effect,” that peaked approximately 400 milliseconds after the word was revealed. But how to interpret it remained unclear. Was the brain reacting because the word’s meaning was nonsensical in the context of the sentence? Or might it have been reacting because the word was simply unanticipated, violating whatever predictions the brain had made about what to expect?
In 2005, Kutas and her team conducted another study that pointed to the latter possibility. People were again asked to read a sentence one word at a time on a screen: “The day was breezy so the boy went outside to fly ____.” Because “a kite” seemed the most likely way to finish the sentence, the subjects expected to see “a” next, a word that had no intrinsic meaning but did predict the word to follow. When the participants saw “an” instead (as in “an airplane”), they experienced an N400 effect, seemingly because the brain had to process a mismatch between its expectation and reality. The effect was apparently unrelated to the meaning of the word or any difficulty in processing the presented stimulus itself.
The 2005 finding seemed like a great fit for the predictive coding framework. But in April 2018, a paper published in eLife reported that several labs have been unable to replicate the result. Now, other researchers have started to respond, some claiming that subtleties in the replication methods still favor the prediction-based interpretation.
This back-and-forth reflects much of the debate that’s surrounded predictive coding. Experiments like Kutas’ are subject to many interpretations. They can be explained by models other than predictive coding, and they fall short of definitive proof of the hypothesis because they don’t delve into the actual mechanisms at play. While the idea that the brain is constantly making inferences (and comparing them to reality) is fairly well-established at this point, proponents of predictive coding have been seeking ways to prove that their particular version of the story is the right one — and that it extends to all of cognition.
Bayesian Brains and Efficient Computing
The foundational insight that the brain perpetually makes and evaluates its own predictions about ongoing experiences wasn’t always taken for granted. The view of neuroscience that dominated the 20th century characterized the brain’s function as that of a feature detector: It registers the presence of a stimulus, processes it, and then sends signals to produce a behavioral response. Activity in specific cells reflects the presence or absence of stimuli in the physical world. Some neurons in the visual cortex, for instance, respond to the edges of objects in view; others fire to indicate the objects’ orientation, coloring or shading.
But the process turned out to be far less straightforward than it seemed. Further tests found that as the brain perceives, say, a longer and longer line, the detector neurons for lines stop firing even though the line hasn’t disappeared. And the fact that so much information seemed to be communicated through mysterious top-down feedback connections suggested that something else was going on.
That’s where the “Bayesian brain” comes into play, a general framework with roots dating back to the 1860s that flips the traditional model on its head. The theory proposes that the brain makes probabilistic inferences about the world based on an internal model, essentially calculating a “best guess” about how to interpret what it’s perceiving (in line with the rules of Bayesian statistics, which quantifies the probability of an event based on relevant information gleaned from prior experiences). Rather than waiting for sensory information to drive cognition, the brain is always actively constructing hypotheses about how the world works and using them to explain experiences and fill in missing data. That’s why, according to some experts, we might think of perception as “controlled hallucination.”
In that vein, the Bayesian brain also explains why visual illusions work: Two dots blinking in rapid alternation on a screen, for example, look like a single dot moving back and forth, so our brains unconsciously start to treat them like a single object. Understanding how objects move is a higher-level type of knowledge, but it fundamentally influences how we perceive. The brain is simply filling in gaps in information — in this case, about motion — to paint a picture that’s not entirely accurate.
But in spite of the clear role that generative models and expectations play in brain function, scientists have yet to pinpoint exactly how that’s implemented at the level of neural circuits. “The Bayesian brain story is relatively agnostic about what the underlying mechanisms are,” said Mark Sprevak, a professor of philosophy of mind at the University of Edinburgh in Scotland.
Enter predictive coding theory, which offers specific formulations of how brains can be Bayesian. Predictive coding gets its name from a technique for transmitting telecommunications signals more efficiently: Because video files contain a lot of redundancy from one frame to the next, it’s inefficient to encode every pixel in every image when compressing the data. Instead, it makes more sense to encode the differences between adjacent frames and then work backward to interpret the entire video.
In 1982, scientists found that this idea has a neat application in neuroscience — because it appears to explain how neurons in the retina encode information about a visual stimulus and transmit it along the optic nerve. It’s also been cemented as a principle of how the brain’s reward system functions: Dopamine neurons encode the magnitude of the mismatch between an expected reward and the actual reward that’s received. These prediction errors, researchers say, help animals update their future expectations and drive decision-making.
But despite these examples, scientists mostly saw predictive coding as a process specific to certain networks. Functional magnetic resonance imaging tests and other types of experiments have begun to change that.
A Universal Framework
Part of what makes the predictive coding hypothesis so compelling is its incredible explanatory power. “What I find convincing is how so many things all get accounted for under this story,” said Andy Clark, a professor of logic and metaphysics at the University of Edinburgh and an expert on the theory.
First, it unifies perception and motor control under a single computational process. The two are essentially opposite sides of the same coin: In each case, the brain minimizes prediction errors, but in different ways. With perception, it’s the internal model that gets adjusted; with motor control, it’s the actual environment. (For the latter, imagine that you want to raise your hand. If your hand is not already raised, that discrepancy generates a large prediction error — which can be minimized if you simply move your hand.)
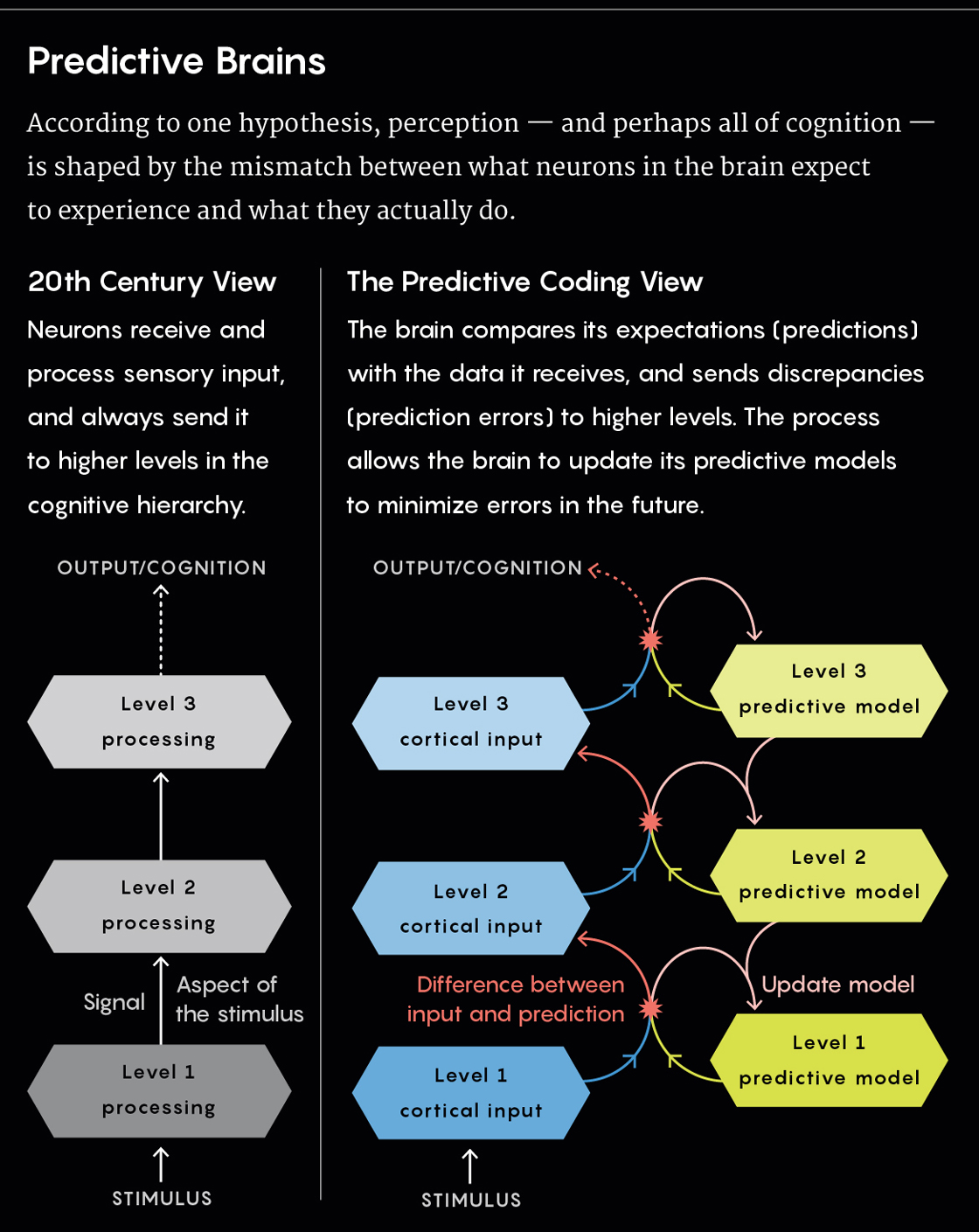
Credit: Lucy Reading-Ikkanda/Quanta Magazine.
Experiments in perception and motor control have so far provided the strongest evidence for predictive coding theory. In a 2018 paper published in the Journal of Neuroscience, for example, experimenters had subjects read the word “kick” on a screen, then had them listen to a distorted recording of the word “pick” that sounded like a loud whisper. Many heard “kick” instead, and fMRI scans revealed that the brain represented the initial “k” or “p” sound most strongly — the sound that correlated to a prediction error. If the brain were simply representing its perceptual experience, the strongest signal should have corresponded to “ick” instead (because that was represented both on screen and in the audio).
But efforts are also ongoing to widen predictive coding’s relevance beyond perception and motion — to establish it as the common currency of everything going on in the brain. “It’s like having building blocks with which different strategies can be built,” Clark said. Different brain regions simply trade in different kinds of prediction.
Friston, among others, claims this applies to higher cognitive processes including attention and decision-making. Recent computational work on the prefrontal cortex has implicated predictive coding in working memory and goal-directed behaviors. Some researchers theorize that emotions and moods can be formulated in predictive coding terms: Emotions could be states the brain represents to minimize prediction error about internal signals such as body temperature, heart rate or blood pressure. If the brain recognizes that it’s agitated, for instance, then it knows all those factors are going up. Perhaps that’s also how the concept of selfhood can emerge.
Most of the work being done in this vein focuses on how predictive coding might explain neuropsychiatric and developmental disorders. “The idea,” Friston said, “is that if the brain is an inference machine, an organ of statistics, then when it goes wrong, it’ll make the same sorts of mistakes a statistician will make.” That is, it will make the wrong inferences by placing too much or too little emphasis on either predictions or prediction errors.
Aspects of autism, for instance, might be characterized by an inability to ignore prediction errors relating to sensory signals at the lowest levels of the brain’s processing hierarchy. That could lead to a preoccupation with sensations, a need for repetition and predictability, sensitivity to certain illusions, and other effects. The reverse might be true in conditions that are associated with hallucinations, like schizophrenia: The brain may pay too much attention to its own predictions about what is going on and not enough to sensory information that contradicts those predictions. (Experts are quick to caution, however, that autism and schizophrenia are much too complicated to be reduced to a single explanation or mechanism.)
“The most profound part of it is that it shows us how vulnerable our mental function is,” said Philip Corlett, a clinical neuroscientist at the Yale School of Medicine. Experiments in Corlett’s lab set up new “beliefs” in healthy subjects that encourage them to hallucinate stimuli they previously experienced. (For instance, in one experiment, the scientists conditioned participants to associate a tone with a visual pattern. The subjects continued to hear the tone when they saw the pattern, even when there was no sound at all.) The researchers are trying to unravel how those beliefs translate into perception. Through these studies, “we’ve got evidence suggesting that perception and cognition are not that separate,” Corlett said. “New beliefs can be taught and can change what you perceive.”
But that evidence hasn’t come close to offering proof — until now.
Zooming in for a Better Look
“Experimental work often shows a particular result is compatible with predictive processing, but not that it’s the best explanation of that result,” Sprevak said. The theory is widely accepted in the cognitive sciences, but “in the field of systems neuroscience, it’s still a bit of an underdog,” said Georg Keller, a neuroscientist at the Friedrich Miescher Institute for Biomedical Research in Switzerland. His lab is trying to change this with harder evidence.
In a 2017 study published in Neuron, Keller and his colleagues observed the emergence of neurons in the visual system of mice that became predictive over time. It began with an accident, when they set out to train the mice on a video game, only to find that the virtual world had gotten its directions mixed up. Ordinarily — and up until the time of the experiment — the mice saw their field of vision move to the right whenever they turned to the left, and vice versa. But someone had unintentionally flipped the virtual world the researchers used in the study, inverting left and right so that turning leftward meant the mice also experienced vision leftward. The researchers realized that they could capitalize on the accident. They monitored the brain signals that represented this visual flow and found that the signals changed slowly as the mice learned the rules of the inverted environment. “The signals looked like predictions of visual flow to the left,” Keller said.
If the signals had simply been sensory representations of the mouse’s visual experience, they would have flipped immediately in the virtual world. If they had been motor signals, they wouldn’t have flipped at all. Instead, “it is about identifying prediction,” Keller said. “The prediction of visual flow, given movement.”
“The work provides a kind of evidence that didn’t exist before,” Clark said. “A very local, cell-by-cell, layer-by-layer demonstration that the best-fit model for what’s going on is predictive coding.”
Similar findings in the parts of the brain that macaques use to process faces were reported around the same time. Previous work had already shown that neurons at lower levels in the network code for orientation-based aspects of a face — by firing at, say, any face in profile. At higher levels, neurons represent the face more abstractly, by paying attention to its identity rather than its position. In the macaque study, the researchers trained monkeys on pairs of faces in which one face, appearing first, always predicted something about the second one. Later, the experimenters interfered with those expectations in specific ways, by showing the same face from a different angle, or an entirely different face. They found prediction errors in lower-level areas of the face processing network, but these errors were associated not with predictions about orientation but with predictions about identity. That is, the errors stemmed from what was going on at higher levels of the system — suggesting that lower levels construct the error signal by comparing incoming perceptions with predictions descending from higher levels.
“It was exciting to find prediction errors, and to find the specific content of predictions, in that system,” said the paper’s lead author, Caspar Schwiedrzik, a neuroscientist at the European Neuroscience Institute Göttingen in Germany.
According to Lucia Melloni, a researcher at the Max Planck Institute for Empirical Aesthetics in Frankfurt, Germany, her group is starting to see results compatible with an explanation of prediction error in neuronal data currently being collected from humans.
A Race to Find More Predictive Machines
Not everyone agrees that the case for predictive coding in the brain is strengthening. Some scientists accept that the theory can explain certain aspects of cognition but reject the idea that it could explain everything. Others don’t concede even that much. To David Heeger, a professor of psychology at New York University, it’s important to make a distinction between “predictive coding,” which he says is about transmitting information efficiently, and “predictive processing,” which he defines as prediction-making over time. “There’s a lot of confusion in the literature because these things have been assumed to all be part of the same soup,” he said. “And that’s not necessarily the case, nor is it necessarily the best way to go forward in studying it.” Other types of Bayesian models, for instance, might provide a more accurate description of brain function under certain circumstances.
What many experts in the field do agree on, however, is that this research has the potential for exciting applications in machine learning. At present, the vast majority of artificial intelligence research does not involve predictive coding, instead focusing on other kinds of algorithms.
But formulating predictive coding architecture in a deep-learning context could bring machines closer to intelligence, Friston argues.
DeepMind’s GQN serves as a good example of that potential. And in 2017, researchers at the University of Sussex even used virtual reality and artificial intelligence technologies that included predictive coding features to create what they called the “Hallucination Machine,” a tool that was able to mimic the altered hallucinatory states typically caused by psychedelic drugs.
Machine learning advances could be used to provide new insights into what’s happening in the brain by comparing how well predictive coding models perform against other techniques. At the very least, introducing predictive coding into artificial systems could significantly improve the intelligence of those machines.
But before that can happen, much work lies ahead. Scientists need to continue the kind of research being done by Keller, Schwiedrzik and others to determine just where, for instance, the brain’s internal representations reside. And it remains to be seen whether similar experiments can substantiate claims for predictive coding in higher cognitive processes.
Predictive coding “is as important to neuroscience as evolution is to biology,” said Lars Muckli, a neurophysiologist at the University of Glasgow who has done extensive work on the theory. But for now, Sprevak noted, “the jury is still out.”
Jordana Cepelewicz is a staff writer at Quanta Magazine who covers biology.