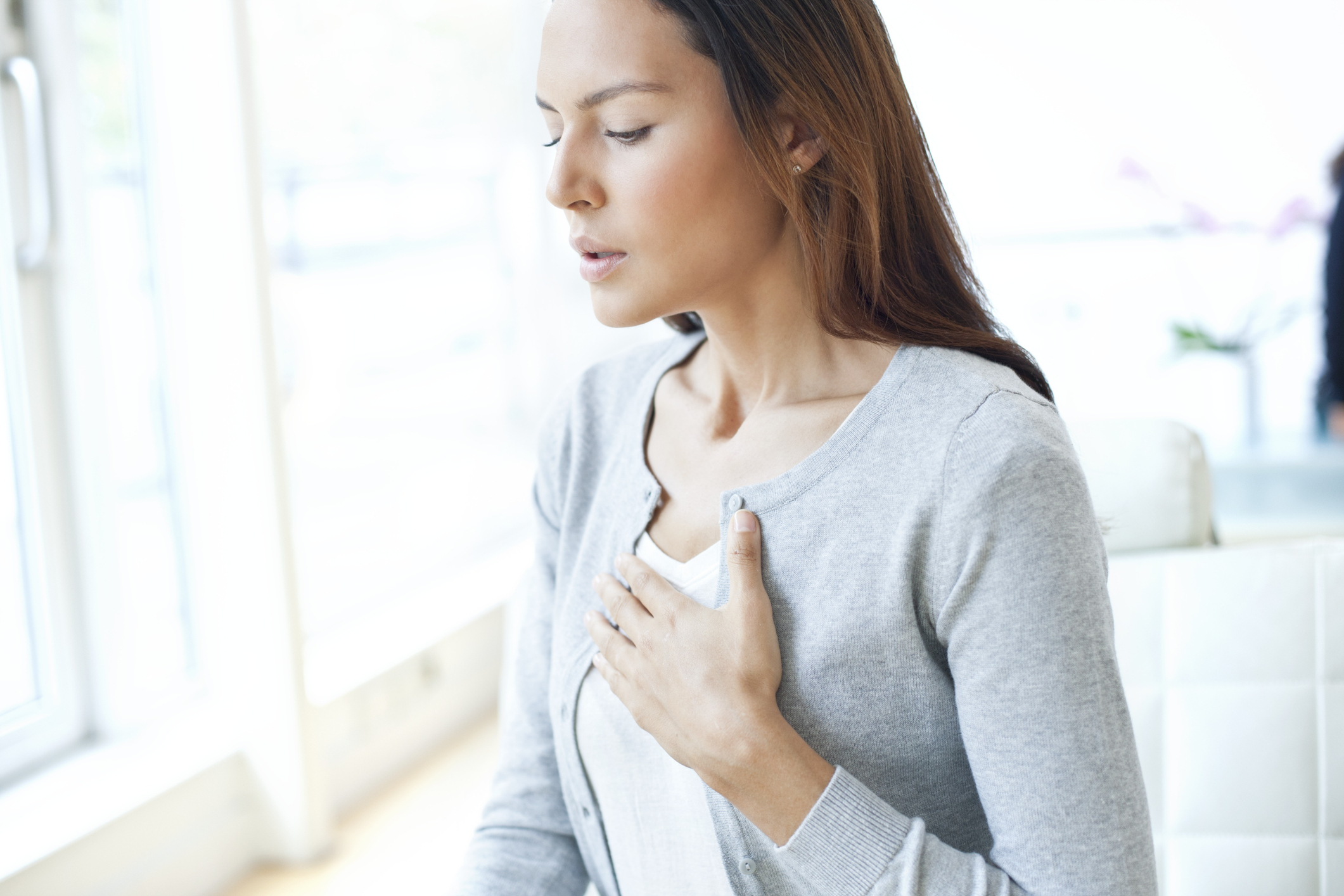
Photo from the Science Photo Library / Getty Images.
Lisa Barlow, whose name I have changed to protect her privacy, is at her kitchen table in Washington DC when she realizes that each Sunday, fifteen passenger trains depart for New Haven, CT. She’s a successful copy editor and has a meeting in New Haven early Monday morning. She has no plans Sunday, so doesn’t care when she arrives or how long it takes. She travels coach so has thirty tickets to choose from: fifteen departures each with two price options.
Should she choose the more-expensive flexible ticket over the locked-in value ticket? Does she want to leave earlier or later? Brunch in DC or lunch in New Haven? She can’t decide.
She scrolls the screen up and down, up and down, faster and faster. Her eyes dart about the webpage. She feels a rising tension in her chest. Her breathing shortens. Her thoughts race in and out of her mind like the breath in her lungs. She touches her face and notices the telltale sign: it’s numb. She reaches into her pocket, where she safeguards a small pill for moments like these. A pharmacologic reset button.
Barlow has had panic attacks since High School—the first over a social drama, the second after her science teacher told her that if she refused to dissect a pig, she’d amount to nothing. She suspects her attacks have something to do with her parents, whose difficult marriage often forced her to choose between them. This, a therapist explained, was an “impossible choice,” one with permanent consequences yet no clear answer. Now as an adult, when faced with a decision that has no clear answer—even something as simple as booking a train ticket—her brain is programmed to panic.
Barlow is a capable and confident professional whose job it is to make hundreds of decisions each day. Why couldn’t she see the difference between which parent to be with and what train to take? There must have been something else going on.
Anxiety as a Learning Problem
Michael Browning is a practicing psychiatrist who directs Oxford’s Computational Psychiatry laboratory. I met Browning as a fourth year medical student. I’d taken a break from Yale to work for six months at Warneford Hospital, a beautiful limestone building from 1826. Browning’s latest paper, Anxious individuals have difficulty learning the causal statistics of aversive environments, had been published in “Nature Neuroscience” just months before I arrived and I was excited to learn cutting-edge neuroscience in the city of dreaming spires.
Browning is a pleasant-looking fellow—normal height, normal build, balding (which I personally hope is normal). My first day, Browning walked me around the lab, introducing me to the other graduate students and post-docs. “This is Daniel Barron, he’s come here from some university in the U.S. to work on some sort of project,” he would say in his Scottish accent with a flat expression. He showed me where the “bog” (bathroom) was, where we had tea, where I could put my cowboy boots and hat if, as a Texan, I felt inclined to wear them on a rainy day.
Although Browning enjoyed cardiology and nephrology as a medical student, he became a computational psychiatrist because he found mental illnesses more compelling. Cardiologists treat the heart like a pump and they measure and calculate how well the pump is working. Nephrologists treat the kidney like a filter and measure and calculate how well the filter is working. Psychiatrists don’t really know how to view the brain. And we don’t know how to measure or calculate how well the brain is working. This is what Browning wants to do.
After his psychiatry residency, Browning began treating patients with major depression and bipolar disorder and noticed how extremely common anxiety is in these patients. Knowing someone has a cognitive symptom—like a panic attack when booking a train ticket—is clinically useful because it suggests a treatment goal (i.e. book tickets without panicking), something to target with clinical interventions like cognitive behavioral therapy (CBT).
CBT helps patients learn to look at anxiety-provoking situations in a new, less threating way. That you can successfully treat anxiety with CBT indicates that CBT is helpful. But on a more fundamental level, it also indicates that patients can learn how to not be anxious. Browning also noted that, reversing this logic, it also means that anxiety involves a learning process gone awry.
Learning on the (Coffee) Run
Measuring how we learn is hard. Experimentally, we can observe people’s behavior; e.g. did someone answer a question correctly? or did they complete a task as they were taught? This treats the brain like a black box, wherein cognitive “stuff” happens and decisions magically appear.
Learning theory provides a way to peer into this black box with mathematics. Learning theory describes how the brain builds models of the world, with the goal of understanding how to behave. According to learning theory, people develop models about the world based on the outcomes of their actions (“I did X and Y happened, so X gives me Y”).
Imagine that one afternoon, you want a really good cup of coffee. To get it, you need to walk to one of two nearby coffee shops: one is an international chain, the other is run by some local hipsters. The chain coffee shop has a lot going for it: there’s a standard menu; the coffee is made nearly the same way every time; the same music plays at corporate-specified volume; the wifi always works. The last time you went, you left with a fairly good cup of coffee and so, in learning theory terms, we’d say this coffee run ended with only a small prediction error, meaning what you expected is roughly what you got. If someone asked you how often this happens, you’d give a ballpark 75 percent of the time because you feel that overall the chain store is a pretty stable, safe bet even if you don’t always leave with a really good cup of coffee.
Now consider the hipster coffee shop: the seasonal menu is locally sourced so your favorite made-from-scratch pastry may or may not be available; rotating “coffee artists” put their own spin on every drink; sometimes there’s jazz, sometimes heavy metal; sometimes the wifi works, often it doesn’t. When the stars align, you have the absolute best coffee experience. But because every few weeks the hipsters change things up, you often leave with no pastry and whatever Lars felt like making you. This leads to a large prediction error and, crucially, you’re never quite sure what to expect. From a learning theory perspective the hipster coffee shop is a volatile environment.
Now imagine that you have three consecutive bad experiences at both places: where do you go next? Because the chain store has a 75 percent overall likelihood of being pretty good, those three strikes don’t affect your belief in the chain store that much and you’re likely to go back. But the hipster coffee shop is more volatile, so after three strikes, you could decide that the place has gone to shit and ne’er return.
In both cases there was a prediction error (although you expected a good cup of coffee, you got a bad one three times), but because the hipster coffee shop is a more volatile place, you weighed the new information more heavily and learned from it more in terms of your overall belief. How much prediction errors sculpt your belief is called the learning rate. Your learning rate for each coffee shop depends on the volatility.
The Brain as a Learning Machine
When he started at Oxford, Browning was keen to measure how people learn. Timothy Behrens and several colleagues had recently designed a reward game wherein participants tried to win a pot of money. To get this money, they had to click on a green or blue rectangle, which would inch them closer (or not) to that money. Because it was unclear which rectangle (blue or green) they needed to choose, players learned which was more likely to lead to a reward by trial and error, while playing the game. Like learning which coffee shop to go to by frequenting different coffee shops multiple times.
Also similar to the coffee shop analogy, Behrens developed two versions of the game: a stable version where blue rectangles led to a reward 75 percent of the time and a volatile version wherein the reward sometimes followed blue, sometimes green. Everyone played both versions of the game, allowing Behrens to see how quickly they could learn each version.
To win, people would have to mentally model how volatile the game was at any point in time. Behrens wanted to see how well the human brain stacked up to an “ideal learner”, or a computer trained to make the winning decision at every step. That seemed like a lot of computational heavy-lifting.
But Behrens discovered—quite surprisingly—that people performed quite well, on par with the ideal learner. Behrens also discovered that he could measure how people played the game differently, depending on how volatile the task was. As the game switched from the steady, 75 percent version to the more volatile version, people adjusted their learning rate in a mathematically-rigorous way. Human brains could actually compute how chain coffee shops differed from hipster coffee shops.
Behrens had created a scenario that allowed him to treat the brain as a decision-making machine. By measuring how someone played his game, Behrens could tell whether someone’s brain was working ideally.
Of course, Browning’s patient’s brains weren’t working ideally. They were anxious. Since he suspected that anxiety was related to learning, he wondered whether he could use Behren’s game to measure where and how his patient’s decision-making machine had broken down.
Measuring is Complex
Browning wanted a measure of how people learn; something tidy that he could discuss with a patient: “Mrs. Robinson, we’re concerned about your learning rate.”
During my medicine rotations, I remember screening patients for known causes of pump malfunction, risk factors of heart disease like hypertension, cholesterol and smoking. There was something deeply triumphant about telling a patient, “you have a low risk of heart disease”, as if we had together avoided catastrophe. This idea of whittling down heart disease to three risk factors is a classic reductionist overture. And it is, by design, a deceit.
Heart disease is complex and, of course, can’t be completely explained by hypertension, cholesterol, and smoking. In fact, we don’t (and might never) understand everything about everyone’s heart disease—genes, exercise, stress from work or love, something in the water, and so on could each play some crucial yet-undefined role. Without question, looking at only three risk factors misrepresents the complexity of heart disease—but, studies have shown that it is a useful simplification.
Clinicians seem comfortable reducing heart disease to three risk factors—we are, after all, only talking about a pump. But we tend to cringe when we consider our inner lives, our own emotions and mental states through the same reductionist lens.
Imagine that you’re Barlow’s psychiatrist. She comes to your office not long after refusing to dissect the pig. She’s shaken and buries her face in her hands crying, “what if I amount to nothing!” You learn about her childhood, her impossible choice between parents. You connect with her, you empathize with her, you want to help.
Now consider your next step: are you going to ask her to sit in front of a computer and click on blue and green rectangles to win a pot of fake money? How much confidence would you have in such a clinical measure? Do you think you could persuade Barlow that her learning rate as measured by the box game has much bearing on her anxiety?
I’d question my devotion to reductionism here too. The box game seems too abstract and too removed from the raw, clinical realities of panic.
But recall that cardiologists once felt this way—the connection between heart attacks, blood pressure and cholesterol isn’t obvious. The very existence of blood pressure wasn’t obvious—even though people had seen blood spurting out of people’s veins for millennia, no one thought to measure blood pressure until the 18th century.
Three hundred years ago, people would have occasional chest pain and then, one day, just drop dead. I wonder what it was like going to a doctor three hundred years ago, worried about this weird, occasional pain you have in your chest. I imagine the doctor would have traced your history: “Tell me more about your pain.” Maybe during this conversation, the doctor would place leeches on your arm to “clean” your blood, maybe he would even cut your arm to get rid of “extra” blood. Without other tools or interventions, your visit was primarily a conversation; a good clinician was probably a good conversationalist. The advent of the stethoscope and sphygmomanometer—both of which require the patient and clinician to be silent—nudged this relationship from dialogue towards data. Perhaps we lost something in that silence: that subtle and artful conversation that took place while the doctor was attaching leeches to your forearm.
Cardiologists didn’t become useful because they thought of cleverer questions to ask their patients, but because they developed tools to reduce complex diseases to things they could measure and study and treat.
Simply figuring out that death had causes—that it wasn’t simply the Fates or Wheel of Fortune—was itself a monumental intellectual leap. Careful investigation reduced death to specific causes like heart attacks caused by heart disease. And it was only decades-long studies of thousands of patients (e.g the Framingham Heart Study) that helped us reduce heart disease from vague, subjective symptoms to specific, measurable risk factors. Data made us comfortable with reductionism because data led us to solutions that matter.
So Browning wants to gather data because he wants to reduce anxiety to a useful measure. Yet reductionism comes at a price. In Barlow’s case, the price might be losing a lot of what is real: her stories. To measure anxiety, we might jettison the richness and complexity of Barlow’s interaction with her mother. But maybe this richness isn’t as important we’d like to believe. No one seems to long for the golden age of “leeching conversation.”
An Anxious Machine
While Behren’s experiment was underway, Browning had begun to work with Sonia Bishop, a computational neuroscientist also at Oxford. Bishop was keen to measure how anxiety affects learning, specifically how anxious people think about future negative events.
Together, they modified Behrens’s reward game—instead of winning money for choosing the correct rectangle, you’d get an electric zap if you choose incorrectly. To see how volatility affected learning rate, they occasionally changed the likelihood of getting shocked. They called this an “aversive learning task” and used it to measure how people with varying levels of anxiety navigate unsavory situations.
They discovered that, like Behren’s original study, non-anxious people could sense when the game was more volatile and adapt their strategy like an “ideal learner”—the more stable the task, the less an unexpected zap affected their beliefs about future events. But the more anxious a person was, the less they recognized and adapted their learning rate during the volatile game. Anxious people, it seemed, were unable to recognize and learn from volatility (which makes me wonder whether hipster coffee shops collect anxious customers).
In their “Nature Neuroscience” paper, Browning and his colleagues wondered whether being cognitively blind to volatility could make the world seem less predictable and negative outcomes less avoidable—perhaps like seeing every unclear decision as one of Barlow’s impossible choices? This in turn might further reinforce someone’s overall level of anxiety, creating a spiral into deepening anxiety and other mental illnesses like depression.
While Browning’s study needs to be extended and replicated, the proposed relationship between volatility and learning rate has clear clinical implications. It reduces clinical focus from cognitive symptoms (e.g. to dissect or not dissect a pig?), to a specific, measurable process that has gone awry. And instead of treating someone’s fear of pig dissection (which is simply one instantiation of an underlying “impossible choice” problem), clinicians could measure how well people perceive and learn from volatility and how this changes with treatment.
The Fire
“So I had a house fire two weeks ago,” Barlow told me when I asked whether previous therapists had measured her anxiety, maybe with clinical symptom scales. Two days before the end of a four month kitchen remodel, her contractor had left a couple of oily rags on the floor. Overnight, some varnish cured and the rags burst into flames. “I lost a bunch of stuff—I probably have those scales, but they might have been ruined.”
“Wait. I’m sorry, what?” I said, taken aback that, after speaking for nearly an hour about her emotional highs and lows, Barlow somehow forgot to tell me that her house had recently caught fire. The same person who was paralyzed by the prospect of buying a train ticket was taking an apartment fire in stride.
“The real damage was from the water and smoke,” she mentioned coolly, “the fire department kind of power washed my apartment.” As she talked, I thought of my own kitchen remodel last year—choosing from the seemingly infinite types of cabinets, knobs, and light fixtures, etc. An impossible number of choices. I mentioned this mountain of decisions and she laughed, “I had a hard time picking out the paint color for the walls and now, I might have to choose where the walls go. But really, no I don’t think I have those measures,” she said, shifting seamlessly back from catastrophe to clinical scales.
Barlow said her therapists typically just ask whether she was feeling better, a question that I often ask my own patients. Every time I do, I’m a bit annoyed with myself—someone with heart disease might walk up a steep flight of stairs and feel a dull pain in their chest, but they certainly wouldn’t feel how much cholesterol was in their blood or how narrow their coronary arteries had become. Clinical tests exist because symptoms rarely reflect the underlying disease process, which is often invisible to us.
Like cholesterol or blood pressure, Browning reminded me that learning rate presents a potential therapeutic target. One could imagine a CBT intervention aimed at helping anxious people better understand volatility in a shifting environment. Or perhaps a medication could modify the brain’s inherent learning rate, allowing someone to better separate “impossible choices” from simple errands. But also like cholesterol or blood pressure, learning rate captures something invisible and unintuitive, something that we’d never see or include in our clinical decision without a tool to measure it.
Perhaps Browning is on to something. Perhaps measuring learning rate could benefit clinical practice. I’m hopeful, but if the history of measuring and treating heart disease is any indication, finding tidy measures of anxiety will take large, collaborative efforts over many years. Measurements of learning are still in the experimental stage, so it’s best to maintain a healthy skepticism, to have a healthy learning rate. “There’s a lot of promise,” Browning cautioned, “What there isn’t is a lot of data.” Hopefully, we’ll have a better answer in a few years.
The views expressed are those of the author(s) and are not necessarily those of Scientific American.