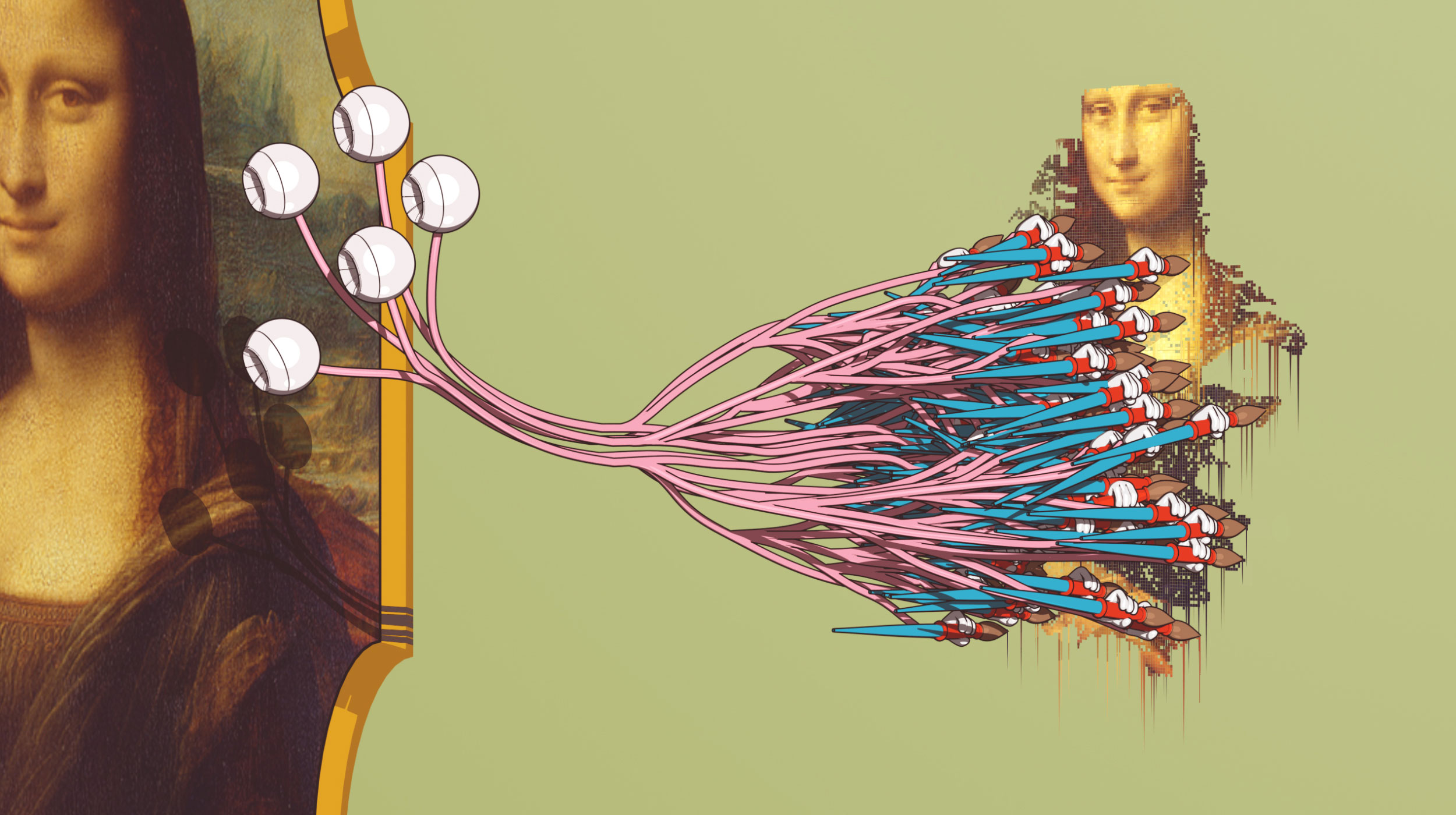
Information from the eye passes through a bottleneck before it gets to the brain’s visual cortex, which heavily processes the sparse signal. Credit: DVDP for Quanta Magazine.
This is the great mystery of human vision: Vivid pictures of the world appear before our mind’s eye, yet the brain’s visual system receives very little information from the world itself. Much of what we “see” we conjure in our heads.
“A lot of the things you think you see you’re actually making up,” said Lai-Sang Young, a mathematician at New York University. “You don’t actually see them.”
Yet the brain must be doing a pretty good job of inventing the visual world, since we don’t routinely bump into doors. Unfortunately, studying anatomy alone doesn’t reveal how the brain makes these images up any more than staring at a car engine would allow you to decipher the laws of thermodynamics.
New research suggests mathematics is the key. For the past few years, Young has been engaged in an unlikely collaboration with her NYU colleagues Robert Shapley, a neuroscientist, and Logan Chariker, a mathematician. They’re creating a single mathematical model that unites years of biological experiments and explains how the brain produces elaborate visual reproductions of the world based on scant visual information.
“The job of the theorist, as I see it, is we take these facts and put them together in a coherent picture,” Young said. “Experimentalists can’t tell you what makes something work.”
Young and her collaborators have been building their model by incorporating one basic element of vision at a time. They’ve explained how neurons in the visual cortex interact to detect the edges of objects and changes in contrast, and now they’re working on explaining how the brain perceives the direction in which objects are moving.
Their work is the first of its kind. Previous efforts to model human vision made wishful assumptions about the architecture of the visual cortex. Young, Shapley and Chariker’s work accepts the demanding, unintuitive biology of the visual cortex as is — and tries to explain how the phenomenon of vision is still possible.
“I think their model is an improvement in that it’s really founded on the real brain anatomy. They want a model that’s biologically correct or plausible,” said Alessandra Angelucci, a neuroscientist at the University of Utah.
Layers and Layers
There are some things we know for sure about vision.
The eye acts as a lens. It receives light from the outside world and projects a scale replica of our visual field onto the retina, which sits in the back of the eye. The retina is connected to the visual cortex, the part of the brain in the back of the head.
However, there’s very little connectivity between the retina and the visual cortex. For a visual area roughly one-quarter the size of a full moon, there are only about 10 nerve cells connecting the retina to the visual cortex. These cells make up the LGN, or lateral geniculate nucleus, the only pathway through which visual information travels from the outside world into the brain.
Not only are LGN cells scarce — they can’t do much either. LGN cells send a pulse to the visual cortex when they detect a change from dark to light, or vice versa, in their tiny section of the visual field. And that’s all. The lighted world bombards the retina with data, but all the brain has to go on is the meager signaling of a tiny collection of LGN cells. To see the world based on so little information is like trying to reconstruct Moby-Dick from notes on a napkin.
“You may think of the brain as taking a photograph of what you see in your visual field,” Young said. “But the brain doesn’t take a picture, the retina does, and the information passed from the retina to the visual cortex is sparse.”
But then the visual cortex goes to work. While the cortex and the retina are connected by relatively few neurons, the cortex itself is dense with nerve cells. For every 10 LGN neurons that snake back from the retina, there are 4,000 neurons in just the initial “input layer” of the visual cortex — and many more in the rest of it. This discrepancy suggests that the brain heavily processes the little visual data it does receive.
“The visual cortex has a mind of its own,” Shapley said.
For researchers like Young, Shapley and Chariker, the challenge is deciphering what goes on in that mind.
Visual Loops
The neural anatomy of vision is provocative. Like a slight person lifting a massive weight, it calls out for an explanation: How does it do so much with so little?
Young, Shapley and Chariker are not the first to try and answer that question with a mathematical model. But all previous efforts assumed that more information travels between the retina and the cortex — an assumption that would make the visual cortex’s response to stimuli easier to explain.
“People hadn’t taken seriously what the biology was saying in a computational model,” Shapley said.
Mathematicians have a long, successful history of modeling changing phenomena, from the movement of billiard balls to the evolution of space-time. These are examples of “dynamical systems” — systems that evolve over time according to fixed rules. Interactions between neurons firing in the brain are also an example of a dynamical system — albeit one that’s especially subtle and hard to pin down in a definable list of rules.
LGN cells send the cortex a train of electrical impulses one-tenth of a volt in magnitude and one millisecond in duration, setting off a cascade of neuron interactions. The rules that govern these interactions are “infinitely more complicated” than the rules that govern interactions in more familiar physical systems, Young said.
Individual neurons receive signals from hundreds of other neurons simultaneously. Some of these signals encourage the neuron to fire. Others restrain it. As a neuron receives electrical pulses from these excitatory and inhibitory neurons, the voltage across its membrane fluctuates. It only fires when that voltage (its “membrane potential”) exceeds a certain threshold. It’s nearly impossible to predict when that will happen.
“If you watch a single neuron’s membrane potential, it’s fluctuating wildly up and down,” Young said. “There’s no way to tell exactly when it’s going to fire.”
The situation is even more complicated than that. Those hundreds of neurons connected to your single neuron? Each of those is receiving signals from hundreds of other neurons. The visual cortex is a swirling play of feedback loop upon feedback loop.
“The problem with this thing is there are a lot of moving parts. That’s what makes it difficult,” Shapley said.
Earlier models of the visual cortex ignored this feature. They assumed that information flows just one way: from the front of the eye to the retina and into the cortex until voilà, vision appears at the end, as neat as a widget coming off a conveyor belt. These “feed forward” models were easier to create, but they ignored the plain implications of the anatomy of the cortex — which suggested “feedback” loops had to be a big part of the story.
“Feedback loops are really hard to deal with because the information keeps coming back and changes you, it keeps coming back and affecting you,” Young said. “This is something that almost no model deals with, and it’s everywhere in the brain.”
In their initial 2016 paper, Young, Shapley and Chariker began to try and take these feedback loops seriously. Their model’s feedback loops introduced something like the butterfly effect: Small changes in the signal from the LGN were amplified as they ran through one feedback loop after another in a process known as “recurrent excitation” that resulted in large changes in the visual representation produced by the model in the end.
Young, Shapley and Chariker demonstrated that their feedback-rich model was able to reproduce the orientation of edges in objects — from vertical to horizontal and everything in between — based on only slight changes in the weak LGN input coming into the model.
“[They showed] that you can generate all orientations in the visual world using just a few neurons connecting to other neurons,” Angelucci said.
Vision is much more than edge detection, though, and the 2016 paper was just a start. The next challenge was to incorporate additional elements of vision into their model without losing the one element they’d already figured out.
“If a model is doing something right, the same model should be able to do different things together,” Young said. “Your brain is still the same brain, yet you can do different things if I show you different circumstances.”
Swarms of Vision
In lab experiments, researchers present primates with simple visual stimuli — black-and-white patterns that vary in terms of contrast or the direction in which they enter the primates’ visual fields. Using electrodes hooked to the primates’ visual cortices, the researchers track the nerve pulses produced in response to the stimuli. A good model should replicate the same kinds of pulses when presented with the same stimuli.
“You know if you show [a primate] some picture, then this is how it reacts,” Young said. “From this information you try to reverse engineer what must be going on inside.”
In 2018, the three researchers published a second paper in which they demonstrated that the same model that can detect edges can also reproduce an overall pattern of pulse activity in the cortex known as the gamma rhythm. (It’s similar to what you see when swarms of fireflies flash in collective patterns.)
They have a third paper under review that explains how the visual cortex perceives changes in contrast. Their explanation involves a mechanism by which excitatory neurons reinforce each other’s activity, an effect like the gathering fervor in a dance party. It’s the type of ratcheting up that’s necessary if the visual cortex is going to create full images from sparse input data.
Currently Young, Shapley and Chariker are working on adding directional sensitivity into their model — which would explain how the visual cortex reconstructs the direction in which objects are moving across your visual field. After that, they’ll start trying to explain how the visual cortex recognizes temporal patterns in visual stimuli. They hope to decipher, for example, why we can perceive the flashes in a blinking traffic light, but we don’t see the frame-by-frame action in a movie.
At that point, they’ll have a simple model for activity in just one of the six layers in the visual cortex — the layer where the brain roughs out the basic outlines of visual impression. Their work doesn’t address the remaining five layers, where more sophisticated visual processing goes on. It also doesn’t say anything about how the visual cortex distinguishes colors, which occurs through an entirely different and more difficult neural pathway.
“I think they still have a long way to go, though this is not to say they’re not doing a good job,” Angelucci said. “It’s complex and it takes time.”
While their model is far from uncovering the full mystery of vision, it is a step in the right direction — the first model to try and decipher vision in a biologically plausible way.
“People hand-waved about that point for a long time,” said Jonathan Victor, a neuroscientist at Cornell University. “Showing you can do it in a model that fits the biology is a real triumph.”
Kevin Hartnett is a senior writer at Quanta Magazine covering mathematics and computer science.