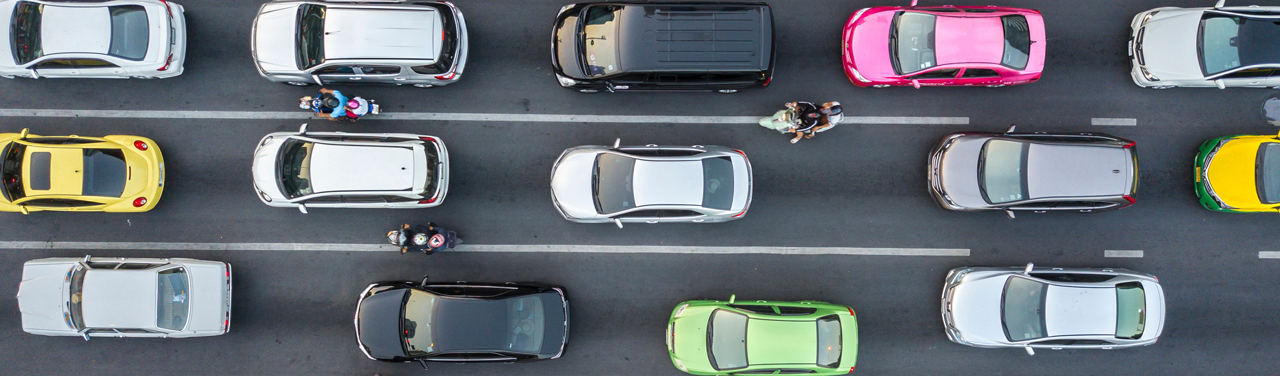
Photo by Raymond Depardon / Magnum Photos.
Few experiences on the road are more perplexing than phantom traffic jams. Most of us have experienced one: The vehicle ahead of you suddenly brakes, forcing you to brake, and making the driver behind you brake. But, soon afterward, you and the cars around you accelerate back to the original speed—and it becomes clear that there were no obstacles on the road, and apparently no cause for the slowdown.
Because traffic quickly resumes its original speed, phantom traffic jams usually don’t cause major delays. But neither are they just minor nuisances. They are hot spots for accidents because they force unexpected braking. And the unsteady driving they cause is not good for your car, causing wear and tear and poor gas mileage.
So what is going on, exactly? To answer this question mathematicians, physicists, and traffic engineers have devised many types of traffic models. For instance, microscopic models resolve the paths of the individual vehicles, and are good at describing vehicle–vehicle interactions. In contrast, macroscopic models describe traffic as a fluid, in which cars are interpreted as fluid particles. They are effective at capturing large-scale phenomena that involve many vehicles. Finally, cellular models divide the road into segments and prescribe rules by which cars move from cell to cell, providing a framework for capturing the uncertainty that is inherent in real traffic.
It soon becomes clear that there were no obstacles on the road, and apparently no cause for the slowdown.
In setting out to understand how a phantom traffic jam forms, we first have to be aware of the many effects present in real traffic that could conceivably contribute to a jam: different types of vehicles and drivers, unpredictable behavior, on- and off-ramps, and lane switching, to name just a few. We might expect that some combination of these effects is necessary to cause a phantom jam. One of the great advantages of studying mathematical models is that these various effects can be turned off in theoretical analysis or computer simulations. This creates a host of identical, predictable drivers on a single-lane highway without any ramps. In other words, your perfect commute home.
Surprisingly, when all these effects are turned off, phantom traffic jams still occur! This observation tells us that phantom jams are not the fault of individual drivers, but result instead from the collective behavior of all drivers on the road. It works like this. Envision a uniform traffic flow: All vehicles are evenly distributed along the highway, and all drive with the same velocity. Under perfect conditions, this ideal traffic flow could persist forever. However, in reality, the flow is constantly exposed to small perturbations: imperfections on the asphalt, tiny hiccups of the engines, half-seconds of driver inattention, and so on. To predict the evolution of this traffic flow, the big question is to decide whether these small perturbations decay, or are amplified.
If they decay, the traffic flow is stable and there are no jams. But if they are amplified, the uniform flow becomes unstable, with small perturbations growing into backwards-traveling waves called “jamitons.” These jamitons can be observed in reality, are visible in various types of models and computer simulations, and have also been reproduced in tightly controlled experiments.
In macroscopic, or “fluid-dynamical,” models, each driver—interpreted as a traffic-fluid particle—observes the local density of traffic around her at any instant in time and accordingly decides on a target velocity: fast, when few cars are nearby, or slow, when the congestion level is high. Then she accelerates or decelerates towards this target velocity. In addition, she anticipates what the traffic will do next. This predictive driving effect is modeled by a “traffic pressure,” which acts in many ways like the pressure in a real fluid.
Phantom jams are not the fault of individual drivers, but result instead from the collective behavior of all drivers on the road.
The mathematical analysis of traffic models reveals that these two are competing effects. The delay before drivers reach their target velocity causes the growth of perturbations, while traffic pressure makes perturbations decay. A uniform flow profile is stable if the anticipation effect dominates, which it does when traffic density is low. The delay effect dominates when traffic densities are high, causing instabilities and, ultimately, phantom jams.
The transition from uniform traffic flow to jamiton-dominated flow is similar to water turning from a liquid state into a gas state. In traffic, this phase transition occurs once traffic density reaches a particular, critical threshold at which the drivers’ anticipation exactly balances the delay effect in their velocity adjustment. The most fascinating aspect of this phase transition is that the character of the traffic changes dramatically while individual drivers do not change their driving behavior at all.
The occurrence of jamiton traffic waves, then, can be explained by phase transition behavior. To think about how to prevent phantom jams, though, we also need to understand the details of the structure of a fully established jamiton. In macroscopic traffic models, jamitons are the mathematical analog of detonation waves, which naturally occur in explosions. All jamitons have a localized region of high traffic density and low vehicle velocity. The transition from high to low speed is extremely abrupt—like a shock wave in a fluid. Vehicles that run into the shock front are forced to brake heavily. After the shock is a “reaction zone,” in which drivers attempt to accelerate back to their original velocity. Finally, at the end of the phantom jam, from the drivers’ perspective, is the “sonic point.”
The name “sonic point” comes from the analogy with detonation waves. In an explosion, it is at this point that the flow turns from supersonic to subsonic. This has crucial implications for the information flow within a detonation wave, as well as in a jamiton. The sonic point provides an information boundary, similar to the event horizon in a black hole: no information from further downstream can affect the jamiton through the sonic point. This makes dispersing jamitons rather difficult—a vehicle can’t affect the jamiton through its driving behavior after passing through.
Instead, the driving behavior of a vehicle must be affected before it runs into a jamiton. Wireless communication between vehicles provides one possibility to achieve this goal, and today’s mathematical models allow us to develop appropriate ways to use tomorrow’s technology. For example, once a vehicle detects a sudden braking event followed by an immediate acceleration, it can broadcast a “jamiton warning” to the vehicles following it within a mile distance. The drivers of those vehicles can then, at the least, prepare for unexpected braking; or, better still, increase their headway so that they can eventually contribute to the dissipation of the traffic wave.
The character of the traffic changes dramatically while individual drivers don’t change their driving behavior
at all.
The insights we glean from fluid-dynamical traffic models can help with many other real-world problems. For example, supply chains exhibit a queuing behavior reminiscent of traffic jams. Jamming, queuing, and wave phenomena can also be observed in gas pipelines, information webs, and flows in biological networks—all of which can be understood as fluid-like flows.
Besides being an important mathematical case study, the phantom traffic jam is, perhaps, also an interesting and instructive social system. Whenever jamitons arise, they are caused by the collective behavior of all drivers—not a few bad apples on the road. Those who drive preventively can dissipate jamitons, and benefit all of the drivers behind them. It is a classic example of the effectiveness of the Golden Rule.
So the next time you are caught in a warrantless, pointless, and spontaneous traffic jam, remember just how much more it is than it seems.
Benjamin Seibold is an Assistant Professor of Mathematics at Temple University.