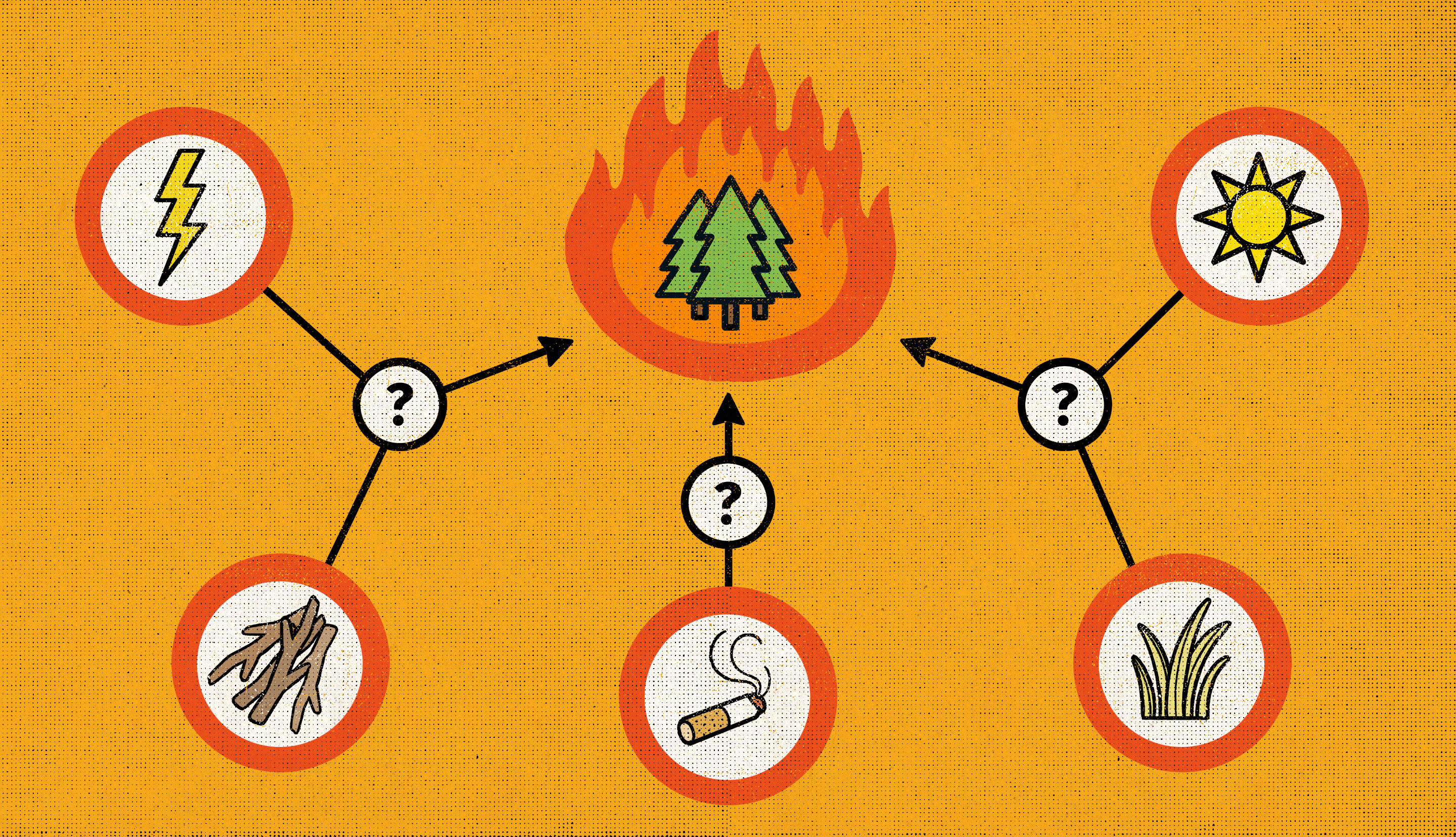
Credit: Dan Page for Quanta Magazine.
You often hear the admonition “correlation does not imply causation.” But what exactly is causation? Unlike correlation, which has a specific mathematical meaning, causation is a slippery concept that has been debated by philosophers for millennia. It seems to get conflated with our intuitions or preconceived notions about what it means to cause something to happen. One common-sense definition might be to say that causation is what connects one prior process or agent — the cause — with another process or state — the effect. This seems reasonable, except that it is useful only when the cause is a single factor, and the connection is clear. But reality is rarely so simple.
Although we tend to credit or blame things on a single major cause, in nature and in science there are almost always multiple factors that have to be exactly right for an event to take place. For example, we might attribute a forest fire to the carelessly thrown cigarette butt, but what about the grassy tract leading to the forest, the dryness of the vegetation, the direction of the wind and so on? All of these factors had to be exactly right for the fire to start. Even though many tossed cigarette butts don’t start fires, we zero in on human actions as causes, ignoring other possibilities, such as sparks from branches rubbing together or lightning strikes, or acts of omission, such as failing to trim the grassy path short of the forest. And we tend to focus on things that can be manipulated: We overlook the direction of the wind because it is not something we can control. Our scientifically incomplete intuitive model of causality is nevertheless very useful in practice, and helps us execute remedial actions when causes are clearly defined. In fact, artificial intelligence pioneer Judea Pearl published a 2018 book about why it is necessary to teach cause and effect to intelligent machines.
However, clearly defined causes may not always exist. Complex, interdependent multifactorial causes arise often in nature and therefore in science. Most scientific disciplines focus on different aspects of causality in a simplified manner. Physicists may talk about causal influences being unable to propagate faster than the speed of light, while evolutionary biologists may discuss proximate and ultimate causes as mentioned in our previous puzzle on triangulation and motion sickness. But such simple situations are rare, especially in biology and the so-called “softer” sciences. In the world of genetics, the complex multifactorial nature of causality was highlighted in a 2018 Quanta article by Veronique Greenwood that described the intertwined effects of genes.
One well-known approach to understanding causality is to separate it into two types: necessary and sufficient. If 2 cannot be caused unless 1 is present, then 1 is a necessary cause of 2; if the presence of 1 implies the occurrence of 2, then 1 is a sufficient cause. Note how these definitions leave the door open for other causative factors: 1 may be a necessary cause, but may require other “contributory causes” to make 2 happen. Similarly, 1 may be a sufficient cause, but may not be necessary: 3 may cause 2 as well.
The search for a clear and comprehensive theory of causality may well be a philosophical chimera. However, as Insights readers know, our philosophy is that all subjects, however complicated, can be explored through puzzles. So let’s explore multifactorial causation using some simplified mathematical models, restricting ourselves to just three causative factors and omitting interactions between causative factors over time.
Consider a scenario where there are three causative factors, a, b and c, which are real variables that take values between 0 and 2. The three factors interact together to determine the value of a hidden factor, d. If the value of d is within a certain window, then a particular event occurs (Y). If not, the event fails to occur (N).
Problem 1
Consider three models of causation: i) a simple linear interaction between a, b and c (where the value of d is the sum of the three after each is multiplied by its own nonzero constant factor); ii) a “tennis serve” model where a, b and c are like the height, vertical and lateral angle of the hit and d is the position where the ball lands, which has be in the service court; and iii) a “genetic model” where a, b and c are gene products, two of which interact multiplicatively to form an intermediate product that interacts linearly with the third gene product to determine the final concentration of d. The size of the window for d to result in the target event can be set arbitrarily, but must be less than one-twentieth of the total range of values that d can take as a, b and c vary between their extreme values.
Which of the three models described can allow the target event to occur only when a, b and c are all greater than 1 or are all less than or equal to 1, but in no other circumstance? Can you think of a way that a, b and c can interact that will naturally give rise to this result?
The required result is shown in the table below. A “Y” in a specific cell means that the target event can occur somewhere within the sub-ranges of a, b and c values specified in the particular cell; an “N” means that it cannot.
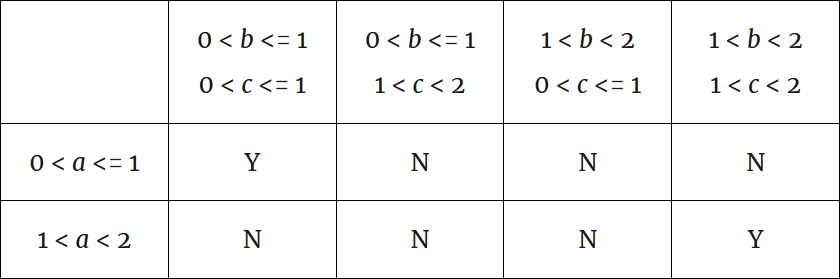
Problem 2
What are the maximum number of cells in each of these models that can show a “Y”?
Problem 3
Of the 256 possible Y-N patterns that these tables can contain, which model can achieve the most? Can it achieve all?
Of course, these causative models are still quite simple, but hopefully they show how complicated multifactorial causality can get in the real world. When that happens, our simplistic compulsion to find a single cause or agent can lead us to invent spurious causes. I invite readers to cite examples of this phenomenon.
Happy puzzling!
The solution has been published here.
Pradeep Mutalik is a medical research scientist at the Yale Center for Medical Informatics and a lifelong puzzle enthusiast.